As business data and analytics professionals, we thrive on crunching, modeling, and interpreting data to support business objectives. With so many data points to consider (and our passion for analysis), it’s easy to go down the rabbit hole to chase down every outlier, eliminate every possible inaccuracy, and project for every probable outcome. From personal experience, we know that this rabbit hole can be bottomless – which begs the question: “How good is good enough?”
Related Content: Maximize ROI With Agile Business Intelligence
# When Does Data Analysis Become Paralysis?
To the data enthusiasts, we know how enticing it is to delve deep into your analysis. While this might illuminate new business insights, it can also give you too much
information for the job. Don’t believe us? Let’s take a quick trip…
The word "inch" in various languages comes from their word for "thumb." The first definition, given by King David I of Scotland, was the width of a man's thumb. However, this wasn't scientifically accurate due to varying thumb widths. Over time, it has been redefined, and now, an inch equals precisely 25.4 millimeters. But what's a millimeter? It's one-thousandth of a meter. And a meter? It's the distance light travels in a vacuum in 1/299792458th of a second. And for those curious: a second is the length of time it takes the electrons in the cesium 133 atom to vibrate 9,192,631,770 times.
Now, suppose you are building a house. You have two tools for measurement – a complex device that measures length based on the cesium-lightspeed definition of the inch and a standard tape measure where an inch is about your thumb's width. Which would you use? Would your choice change if you were building a bookcase or a spaceship?
# Understanding Data Analytics for Business Intelligence
Business data and analytics professionals often lean towards precision, akin to using the cesium-lightspeed definition of an inch for every project regardless of size, complexity, or deadline. In data, this looks like iterating regression models to get marginal gains in R-squared values, conducting excessive data validation checks, and hunting down even the smallest inconsistencies and outliers. It is easy to go down these rabbit holes because, for those of us who love working with data, this is what we’ve trained for!
However, let’s not forget the ultimate goal: the data we process, model, and decipher is meant to guide business goals. As data analysts, we’re the vital conduit of information that helps businesses make data-driven decisions. Instead of using the cesium-lightspeed definition of an inch, we encourage you to consider the possibility that estimating with your thumb is good enough.
When we ask the question, "How good is good enough?” we focus on our problem statement, move quicker to delivering insights, and encourage thoughtful error analysis.
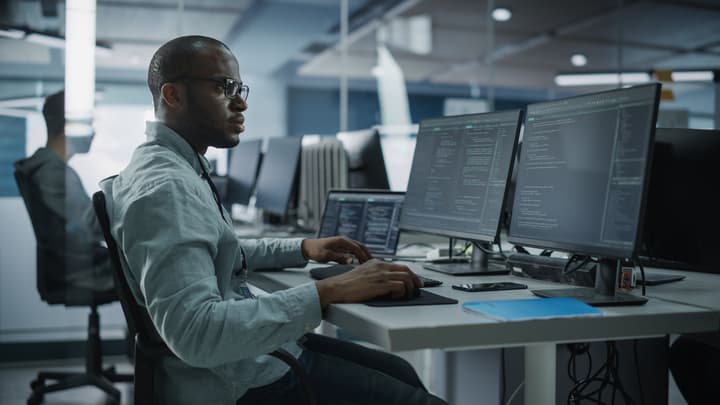
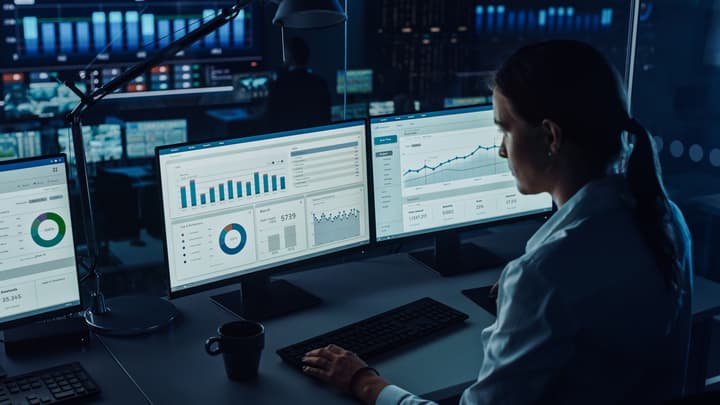
# 3 Considerations For Making Data Analysis More Efficient
Accurate data analysis is a driving factor in making the right business decisions in the short- and long-term. But, efficient analysis is equally important, as fast, data-driven decision-making is a key factor in fostering growth. So, how can leaders and data analysts collaborate to expedite analysis without compromising its quality? Let’s explore three methods.
# 1. Determine The Precision Required For Business Goals
Production environments are a clear example of how questioning the level of precision can keep an analysis focused on the business objective. Supply chain analysts often face the question, “How many machines are required to meet the customer demand?”
Engineers tend to jump into data crunching and mathematical modeling, spending countless hours verifying the source data and validating each detail of the model. But does this answer the question the business needs answered? Perhaps the question is not “How much fractional capacity will be left at this given demand level?” but instead, “Will we need more than five machines by quarter three?”
By taking a step back to truly understand the ask, analysis can redefine their level of precision to deliver data-driven answers that get at the core of the business need.
Questions Leaders Can Ask:
How wrong can we be and still come to the same conclusion for the business?
# 2. Consider The Data Preparation Required
According to a 2020 McKinsey study, “Fast organizations appear to outperform others on innovation, growth, and other metrics” and “…slow decision making is the factor that most strongly separates slower organizations from faster ones.”
As data-driven decision-making continues to show its value, the data analysis driving decisions has to keep pace in order for an organization to stay innovative and competitive.
It’s well known that data preparation accounts for about 80% of the work of data scientists. If data analysts are spending 80% of their time prepping data, then that means that cutting time in this area has the biggest impact. It’s not reducing the quality of an analysis – it’s being more thoughtful about what data is worth scrubbing.
Related Content: Are You Adding To the Data Collection Burden?
Questions Leaders Can Ask:
What if I told you that we will need to do this analysis every month, and our business strategy inputs will keep changing?
What are the requirements to complete this analysis? What are the nice-to-haves?
# 3. Identify the Critical Factors
When we start an analysis by questioning how good is good enough, we’re forced to think carefully through all the factors that influence an analysis. Which of the factors will have the most influence over the business decision? Is there a data-driven method to identify these critical factors?
With big data, not only do we have innumerable amounts of data to leverage to make business decisions, but there is also a lot of noise. Setting the foundation of an analysis to hone in on the factors that move the needle the most means we’re working smarter to produce a high-quality analysis.
Questions Leaders Can Ask:
What assumptions can we make and what are their potential impacts?
What do the experts think of the critical factors you have identified? Do they align with their experiences?
# Conclusion
Efficiently leveraging data to make decisions and influence strategy is critical for organizations to stay competitive. Although high levels of precision in data analyses are more possible than ever, there is merit in going through the exercise of identifying how precise an analysis truly needs to be to reach a business objective. Not sure where to start? Our team of data analytics consultants is here to help you determine how good is good enough, focus your analyses on the decision that needs to be made, move efficiently through analyses, and improve the quality of the work with a deeper foundational understanding.