After nearly a decade of analytics, I’ve noticed a common unintentional trend across a wide range of companies and industries: high data collection burden. In today’s world of big data, this is a multifaceted problem. Describing ‘data collection burden’ can be tricky, so here are two recent client examples:
Example 1: A core business process had 160 fields that were mandatory. As expected, data quality was poor in spite of the growing number of data quality employees that were constantly following up with their business teams to address the gap.
Example 2: An internal survey, sent to a random sample of employees, included this intro text: ‘This survey only takes a few minutes to complete and you will not be asked to complete this survey again for at least 1 year.’ The survey contained dozens of questions and had a response rate <15%.
In both examples, you can see that the outcome was suboptimal and created extra cycles for the employees. The good news is that you can avoid these stumbling blocks. Let’s unpack how to flip the script and get better results with less work.
# How did we get here?
No one sets out with the intent to design a data element with hundreds of fields or a survey with a low response rate, but nonetheless, it happens. As a business leader, you know your team is busy, but balancing the amount of data you ‘need’ with what your team can handle is a balancing act. Asking for more data can quickly become counterproductive. Here are a handful of scenarios to look for:
— Data isn’t filled out, so the dreaded * (asterisk) is imposed–making fields mandatory.
— Analysts continuously bring you requests for additional fields (or attributes) in your data to ‘support reporting’.
— The marginal cost of each additional request is minimal compared to the overall burden: it’s too easy to justify ‘one more question’ or ‘one more field’ and ignore the overall burden.
— Data is critical to your operations, but with falling quality, it’s easier to add more data quality folks than to critically examine existing data collection practices.
# What’s the cost?
In the age of big data, the pressure to collect more data is high, but the burden of unnecessary data collection is measured in human productivity. It’s an extra load your team drags around on every task, slowing their progress and productivity. When you listen to the clicking of your team’s keyboards, you have to wonder ‘is all that typing really improving the bottom line?’
Bottom line: if data collection burden continues to go unchecked, the costs to you, your team, and your organization begin to mount. Unnecessary burden saps the motivation of your people, lowers their productivity, decreases data quality, and makes it more likely that your employee will burnout and leave...because the painful parts of their job just aren’t worth it.
None of this is good, and most of it is avoidable.
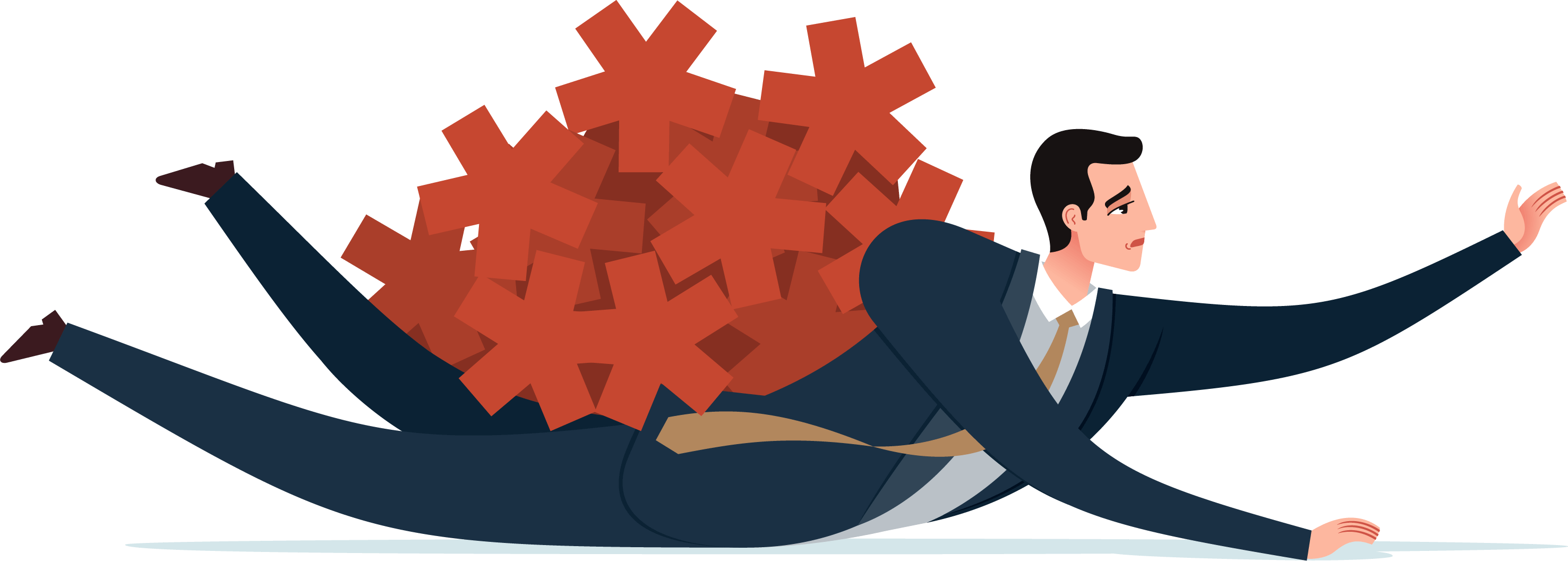
# Alleviate the burden by shifting your data collection mindset
Before you ask your team to manually enter more data, ask yourself these questions:
1. Do you really need this data to satisfy your strategy? You should only aspire to collect data that helps you answer strategic questions.
2. Are you already collecting this somewhere else? In my experience, data is often duplicated because its ‘easier’ to collect it than to connect systems. Don’t fall for this trap: if you need the data, it’s worth sourcing it correctly.
3. Can you get the data automatically? System logs are powerful. Instead of asking your team to enter today’s date on a form, read the form’s metadata and you will have higher accuracy at a lower cost.
4. How much can you ask of your team before you start losing quality? This varies by organization, but you should err on the side of requiring far less data.
5. Can you use a lighter touch and still get meaningful data? For example, using a Net Promoter Score is a great option to capture relatively high fidelity sentiment while creating minimal burden.
# Summary
You don’t have to stay stuck in ruts that lead to data elements with hundreds of attributes—and surveys with low response rates. There is a better way to do it. When my clients express frustration, I often tell them, ‘If it didn’t work last time—don’t try harder, try different.’ This may sound simplistic, but it’s an approach that works. And it can work for you.
Be sure to check out the Data & Analytics page for more insights into how we position our client’s teams for success as they evaluate data, build models, and identify trends to drive better predictions.