Artificial Intelligence (AI) is not new to any industry, but the accelerating pace at which AI systems and capabilities evolve—performing tasks that traditionally require human intelligence and involvement—has brought this topic to the forefront for business leaders.
AI mentions during earnings calls spiked in 2023 and persisted strongly in 2024. Tech companies are pushing AI products and features to the forefront of the platforms, systems, and tools they offer. These products offer substantial potential, but they also carry significant risk for business leaders if not correctly applied. Business leaders must gain a comprehensive understanding of AI’s potential and limitations to develop robust, effective strategies for managing and governing AI.
This blog provides an initial foundation of key AI terms for leaders to build upon, discusses the opportunities and limitations of AI and Machine Learning (ML), and touches on recent developments around tools like ChatGPT, which utilize a subset of ML known as Large Language Models (LLMs) or Generative AI (GenAI).
# What is AI/ML?
Artificial Intelligence is a broad field focused on creating systems capable of tasks like reasoning, problem-solving, and natural language understanding. Machine Learning is a subset of AI that trains algorithms to make predictions or decisions with minimal human intervention. Machine learning operates on data that is known and relatively well-structured, and produces insights beyond traditional BI solutions. Within ML, Large Language Models use vast amounts of text data and deep learning techniques to perform complex language tasks, such as translation, summarization, and conversation. LLMs can synthesize information across unknown or unstructured data sets, and produce summarized insights based on patterns it identifies from assessing the information.
# The Limitations and Opportunities of AI in Business
Understanding the limitations and opportunities of AI in business is crucial for setting a realistic AI strategy and leveraging its full potential. Below, we explore common misconceptions about AI and highlight real-world examples to illustrate both its capabilities and its boundaries.
# 1. AI For Human Interactions
- Misconception: AI can interact with customers or employees in a way that fully understands and responds to human emotions, effectively replacing human customer service or HR roles.
- The Reality: AI can recognize and respond to certain emotional cues through natural language processing and sentiment analysis, but it lacks genuine empathy and the ability to fully understand the nuances of human emotions. However, it can enhance emotional intelligence in customer service by analyzing customer interactions and providing real-time suggestions to human agents, helping them respond empathetically and effectively, thus improving customer satisfaction.
- Business Example: A company may implement AI for employee support and HR functions, handling routine queries about company policies and benefits, but it will fail to adequately address sensitive issues like personal conflicts or performance feedback. HR professionals are still required to navigate these complex interpersonal situations, providing the necessary empathy and understanding.
# 2. Autonomous AI Usage
- Misconception: Once deployed, an AI system can operate autonomously without human oversight or intervention.
- The Reality: AI systems require ongoing monitoring, maintenance, and occasional human intervention to ensure they function correctly and ethically. They are not infallible and can make mistakes or develop biases that need to be addressed. However, AI can automate routine tasks with minimal supervision, such as data entry, scheduling, and basic customer inquiries, reducing the burden on human employees and allowing them to focus on more complex activities.
- Business Example: In financial services, an AI system might be used for fraud detection. While it can analyze transaction data to identify suspicious activities, human analysts are needed to review flagged transactions and make final decisions.
# 3. AI to Execute Creative Processes
- Misconception: AI can replace human creativity entirely, generating original and innovative content without any human input.
- The Reality: AI can assist in creative processes by generating ideas, suggesting improvements, or automating repetitive tasks, but it lacks the ability to truly innovate or create art with deep meaning and emotional resonance. However, it can augment creative efforts by suggesting design variations and optimizing content for specific audiences based on analysis of successful marketing campaigns and current trends.
- Business Example: A marketing team might use AI to aid in copywriting tasks or design layouts. AI can produce basic versions and variations and generate drafts of advertisement copy or email campaigns based on provided input and target audience data. However, human marketers and designers are needed to craft messages that resonate with target audiences and incorporate brand voice.
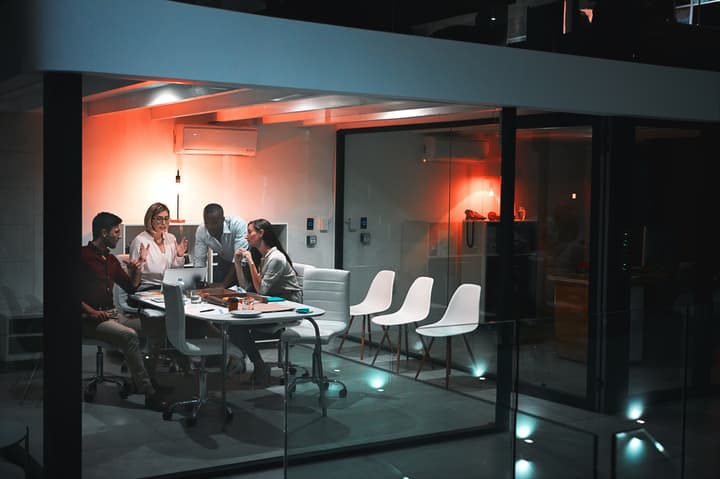
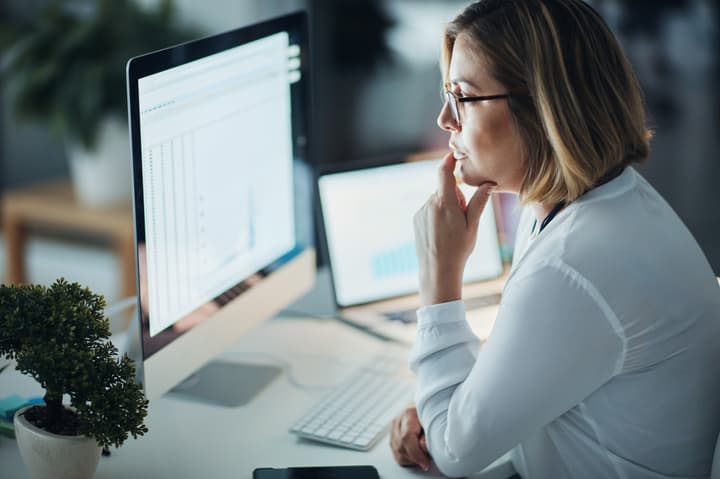
# Leveraging AI in Business
Implementing AI and ML within a business context is a multifaceted process that involves several critical elements. These components work together to ensure that AI tools are not only implemented effectively but also continue to evolve and align with business objectives over time. Here are the key components for using AI in business:
- High-Quality Data: Access to relevant and high-quality data is crucial for training effective ML models. Without sufficient data, the models cannot learn accurately, leading to suboptimal performance and unreliable outcomes. AI should be aimed at problems that can drive the most significant impact, particularly those involving summarizing or deriving insights from large amounts of data, which typical BI solutions struggle with.
- Skilled Personnel: Implementation requires a team of skilled professionals knowledgeable in AI and ML technologies. These individuals are essential for designing, deploying, and maintaining sophisticated AI systems. They play a key role in selecting and building appropriate algorithms or leveraging existing AI tool platforms to realize insights.
- Computational Resources: Adequate hardware and software resources are necessary to support the intensive computations of ML models. Powerful computational infrastructure ensures that models can be trained efficiently and deployed quickly.
- Clear Objectives and Strategy: Starting with a well-defined strategy and clear objectives is essential for guiding AI implementation. This helps align AI initiatives with business goals and measure their impact effectively. Ensuring that the business is ready to support this pace of change is critical, focusing on both people and their processes.
- Continuous Improvement and Monitoring: AI models, particularly LLMs, require ongoing monitoring and tuning to maintain accuracy and align with evolving business needs. Regular updates and adjustments are necessary to adapt to new data and changing conditions. LLMs naturally drift in their responses over short periods if not carefully monitored, so minimizing errors through continuous monitoring and retraining is essential.
- Infrastructure: Robust infrastructure is needed to support continuous model training, evaluation, and deployment. This includes not only physical hardware but also software frameworks and tools that facilitate the development and scaling of AI applications. This also involves optimizing parameters and deploying models for practical use.
- Governance and Compliance: Adherence to ethical and regulatory standards is essential for many industries. Strong governance ensures that ML models remain accurate, scalable, and compliant over time, maintaining the integrity and trustworthiness of AI applications.
Related Content: Interested how top business are shaping their AI strategy?
# Understanding Capabilities & Benefits of AI in Business
Is AI the right fit for your needs? AI and ML solutions are not universally applicable; each is designed to address distinct types of problems at varying scales. It's crucial to evaluate if an AI solution is essential and appropriate for the specific challenges you face.
Capabilities of AI for Business Intelligence
However, the scope of application varies significantly between different types of solutions. Traditional business intelligence (BI) tools are generally more effective for tasks involving well-structured data, offering faster and more precise capabilities for both operational and ad-hoc reporting with existing datasets. These tools enable quick generation of insightful reports, making them accessible and practical for the majority of businesses.
Pattern Recognition
Transitioning from traditional BI tools to more advanced AI applications, Machine Learning excels in scenarios that require the recognition of patterns, making predictions, or enhancing decision-making from complex, less structured data. ML tools, particularly adept at employing Natural Language Processing (NLP), can transform textual content into summaries or sentiment analyses, thereby extracting insights that are not readily discernible with conventional BI tools. Additionally, MLs can identify patterns or anomalies in data that are crucial for detecting malicious behaviors or systemic failures before they become widespread.
Text Generation & Understanding Human Dialogue
Further deepening the capabilities of AI, Large Language Models (LLMs) are exceptionally proficient at processing and generating human language, which makes them ideal for tasks such as text generation, summarization, and translation. They are particularly effective in creating chatbots and virtual assistants that require the capacity to understand and produce human-like dialogue.
However, a potential drawback of LLMs is their dependence on extensive, high-quality training datasets, which can perpetuate existing biases in the data, potentially leading to skewed or inappropriate outputs. Furthermore, LLMs may struggle with maintaining context in lengthy text passages, occasionally generating responses that are coherent yet factually inaccurate or nonsensical.
# AI and Ethics Issues
As AI technologies become increasingly integrated into business operations, ethical considerations must be at the forefront of any AI strategy. Issues such as data privacy, algorithmic bias, and the potential displacement of jobs require careful consideration. Businesses must ensure that AI systems are transparent, accountable, and aligned with societal values to maintain trust and compliance. Developing ethical guidelines and involving diverse stakeholders in AI development can help mitigate risks and foster responsible AI usage.
# Key Takeaways & What To Read Next
The topic of AI is broad and constantly in our news feeds. Amid the hype, it’s crucial to understand the foundations of what AI is and its capabilities to effectively integrate it into your business strategies. From setting an AI strategy to understanding how to implement it and the technical requirements needed to activate it — our experts are here to support you.