Population health is not a new idea, but its application has evolved with the growth of the U.S. hospital system and changes in the regulatory environment. The idea is relatively simple: aim to understand the health outcomes of a group of individuals. Quantifying those outcomes is less simple, as they are affected by various personal, social, economic, and environmental factors. With the alternative payment models (APMs), the burden of regulatory reporting and requirements surrounding program participation, risk aversion, and stratification have taken front and center stage. Given that the focus of population health efforts is around care delivery and prevention, data is an influential and critical decision-maker.
Related Content: Transforming Healthcare —The Work to be Done
In our era of big data, it would seem that we would have easy access to draw insightful conclusions on trends across and within patient populations. However, PHI and data sharing regulations severely inhibit the ability to conduct this analysis, and many other complexities exist. Other challenges include poor data quality, system integration problems, technology limitations, a lack of facility interconnectivity, and process inadequacies. Health systems are ultimately tasked with creating a cohesive and extensive understanding of cost, utilization, quality, access, and efficacy using a web of technologies, platforms, and processes that generally do not integrate well with one another.
While these problems necessitate change, and entire economies have been developed to respond, the answer is rarely clear and never simple. To achieve the desired outcomes, we have to focus on evaluating the current state and identifying problems, and then roll out a solution. Drawing from our healthcare experience in data analytics and change management, we’ve outlined several high-level recommendations to consider when forming an approach.
Related Content: Propeller's People & Change Practice
getting the most out of data
In a perfect world, organizational data is collected, accessible, and understood to help with decision-making – unfortunately, we rarely see organizations whose data space is this tidy. Data is relatively useless without a framework to understand and utilize it. How can we ensure that we understand the origin, accuracy, and completeness of the data? Or that the data is accessible, has been analyzed in a meaningful way, and that analysis has been communicated effectively to inform change? At Propeller, we have developed a data workflow and best practices to help with an approach to a data framework:
1. Collect
It’s critical to understand data collection processes. Engineer these processes in a way that incentivizes data validity and ensures processes are effectively documented.
2. Manage
Review architecture and integration pathways to ensure data stability and accuracy. Make data accessible by publishing centralized data sources for universal access.
3. Analyze
As data is explored and analyzed, ensure a feedback loop is in place to cleanse and validate the data, and that analysis is conducted close to the analytics customers.
4. Communicate
Communicate and visualize data in a manner that facilitates decisions and encourages action.
Bridging the Gap Through Change Management
Anytime a process, technology, or people transformation is planned, it’s important not to overlook critical details that drive success. In healthcare especially, the complexities of downstream contingencies and the patient experience are critical to understand. The principles of change management are levers to pull and adapt to help bridge the gap of insecurity, doubt, and the unknown. We use a combination of methodologies along with our own experience to craft this approach:
1. Mobilize Resources
As early in the planning stages as possible, take into account who will be tasked with communicating with end-users to make them aware of, understand, and adopt the change.
2. Plan & Prepare
Map out when and how communications will be delivered and training should occur, and how the overall impact of the change will be measured.
3. Implement & Manage
Leverage feedback loops to track the progress against the established benchmarks for success and assess risks.
4. Stabilization
Develop the long-term plan for management and tracking, and how to set that process up to both identify problems and repeat successes.
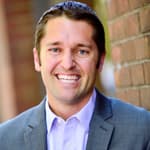
DATA SPECIALIST
Kyle Crawford knows that the best way to lead is by example. His motivation for doing great work not only propels him forward, but also inspires those around him. As an intelligence officer with the United States Marine Corps, Kyle led a platoon of 35 Marines and served as an executive officer for a company of 200 officers. He’s also a data junkie, constantly gathering and analyzing the best intel to make sound decisions and take calculated risks when the odds are in his favor. He holds bachelors degrees from Rensselaer Polytechnic Institute.